In deep learning end-to-end training of segmentation is best
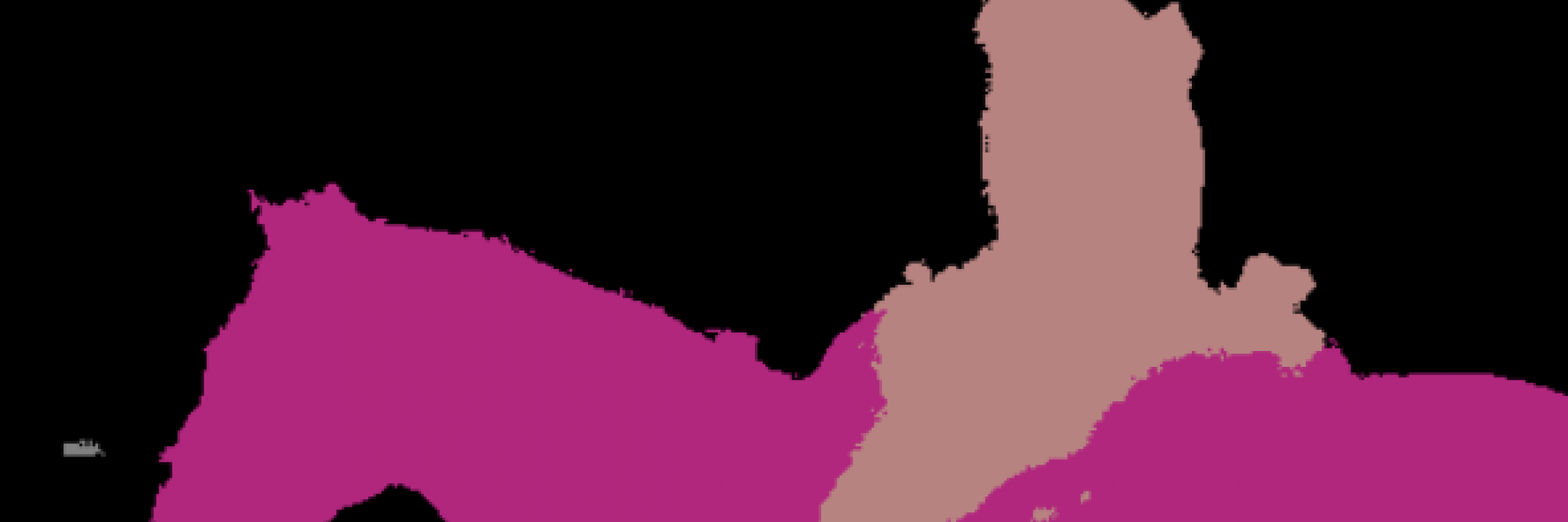
A research team (Dr. Guosheng Lin, Prof. Chunhua Shen, Prof. Ian Reid, Prof. Anton van den Hengel) at the School of Computer Science, The University of Adelaide developed innovative “Deep Structured Learning” techniques that set up the new state-of-the-art semantic image segmentation record in the PASCAL VOC Challenge, which is organised by the University of Oxford. The Adelaide team is the top one currently, outperforming teams from Microsoft Research, Oxford, University of California, Los Angles etc.
Semantic image segmentation is one of the tasks and probably the most challenging one in computer vision and image understanding, which is to label each pixel in images. The Adelaide team teaches a computer how to assign semantic properties to images by using the deep learning technique. The training of the system relies an enormous amounts of labelled exemplars; and takes a few weeks with multiple high-performance Nvidia K40 GPUs.
Deep Learning is the emerging technique behind many companies’ products, among which, Google’s search engine, Facebook’s photo automatic annotation, and iPhone’s Siri are the ones that many of us are using in a daily basis. For many AI-related companies, these days Deep Learning is arguably the most important technique that make the products innovative and drive their business forward.
The PASCAL image challenge’s leader board is at: http://host.robots.ox.ac.uk:8080/leaderboard/displaylb.php?cls=mean&challengeid=11&compid=6&submid=5448
References:
http://arxiv.org/abs/1504.01013, Efficient piecewise training of deep structured models for semantic segmentation
http://arxiv.org/abs/1506.02108, Deeply learning the messages in message passing inference