Improving AI’s understanding of our 3D world
Creating artificial intelligence that can better understand our varied 3D world is one of Matt Howe’s goals.
The Australian Institute for Machine Learning (AIML) PhD student presented a research paper at the International Conference on Digital Image Computing: Techniques and Applications (DICTA 2022) earlier this month that examined how open-source datasets can be used to improve the performance of aerial LiDAR point cloud segmentation models.
LiDAR is a remote sensing technology that uses laser light to measure distances and create high-resolution 3D maps of objects and surfaces. These maps comprise millions of unique points, each referenced in 3D space, called point clouds.
Objects represented in point clouds can be classified using machine learning segmentation models into different types—such as ground surfaces, buildings, vehicles, and vegetation. This is important in a range of point cloud applications related to mapping, surveying, environmental management, and national security.
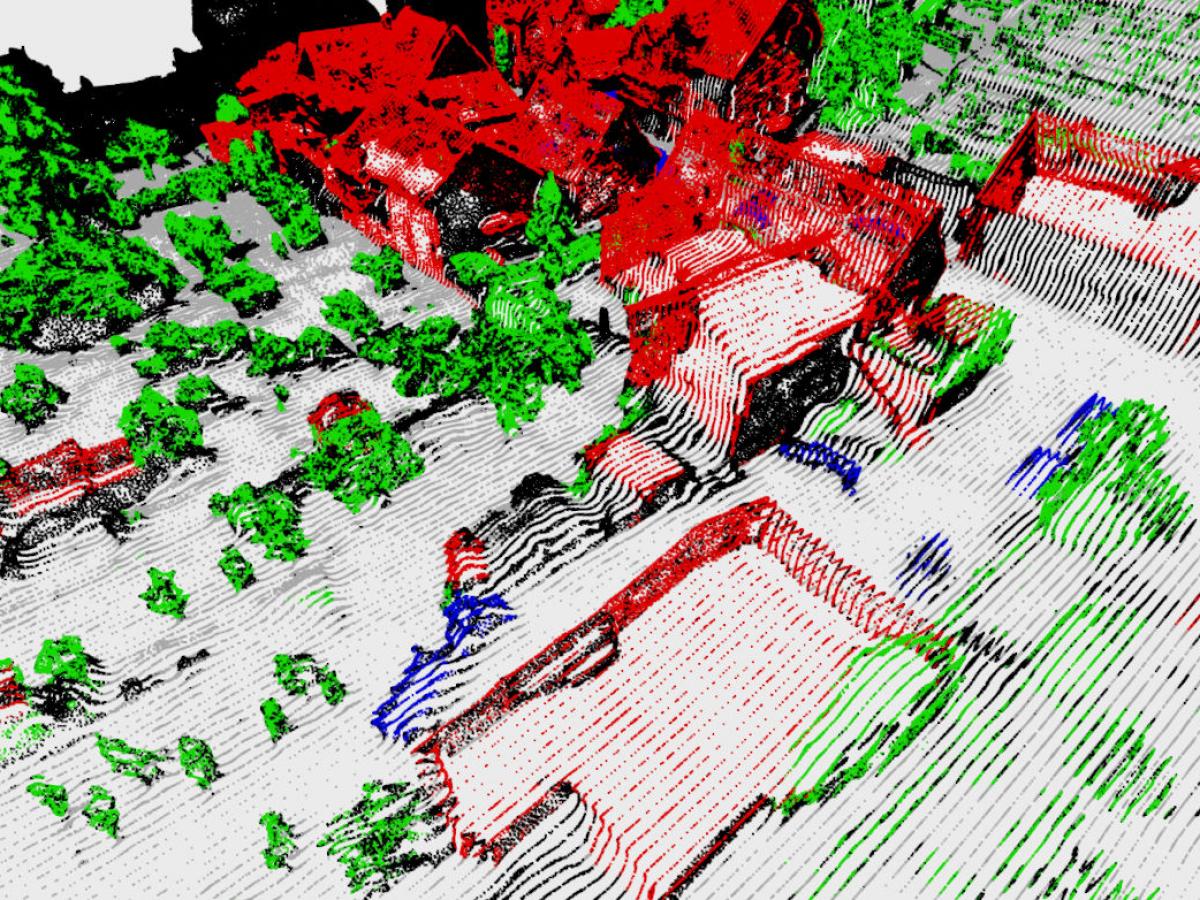
An example of a LIDAR point cloud showing segmentation of different classes of objects; such as buildings (red), trees and vegetation (green), vehicles (blue).
But these segmentation models don’t adapt well to variations across different point cloud data, which can be caused by changing environmental conditions, the sensor type used to collect the point cloud, and where the sensor is mounted (such as a plane, drone, or satellite).
“The problem we’re trying to solve is that models don’t generalise well if you train it on one dataset, and then test it on another one, it fails quite miserably,” Howe said.
The paper—by Howe, and co-authors Boris Repasky (University of Adelaide; Lockheed Martin Australia) and Timothy Payne (Lockheed Martin Australia)—demonstrates a method to improve the performance of point cloud models across different LiDAR data types; machine learning researchers call this generalisation performance.
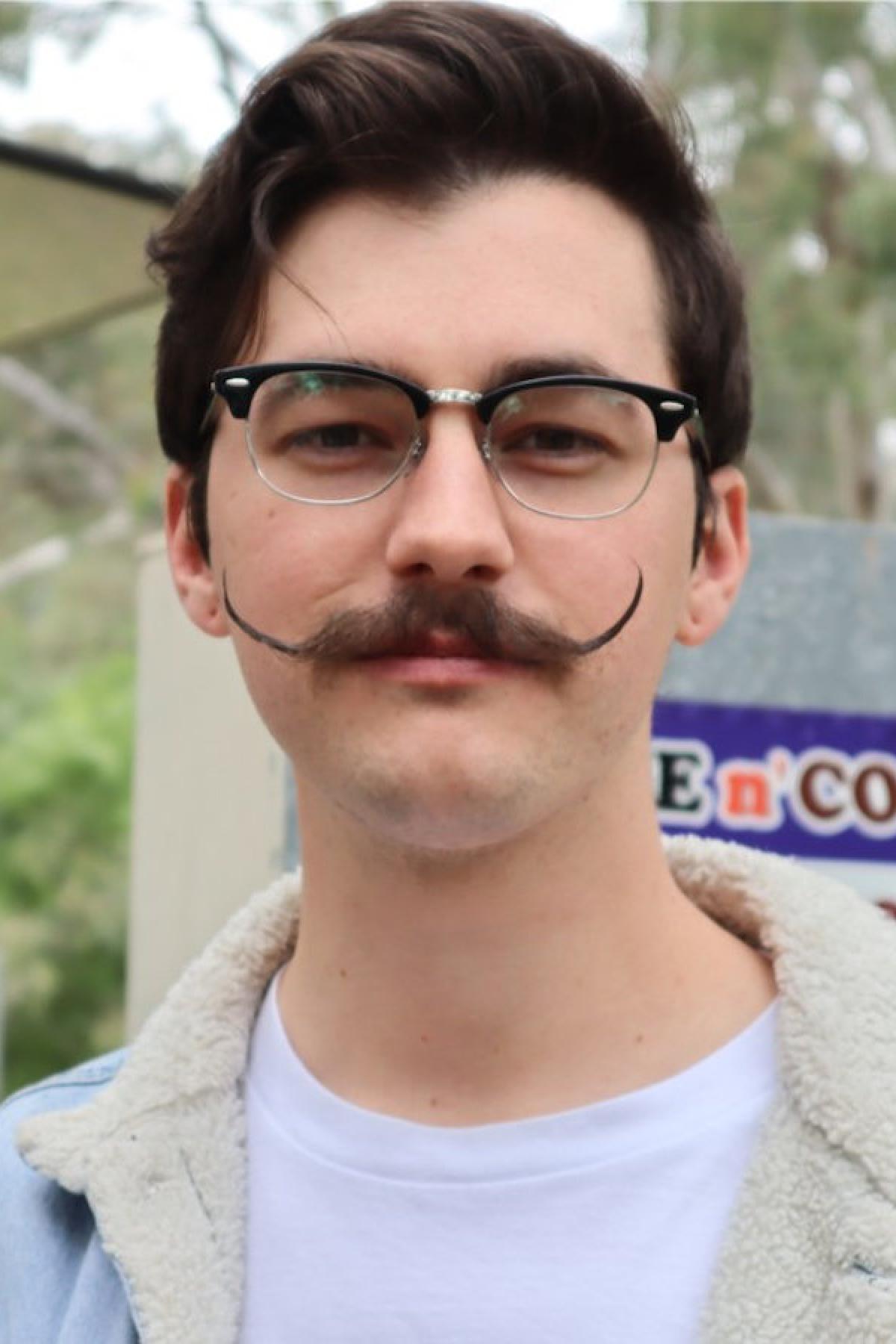
AIML PhD student Matt Howe.
“Let's say we take a LiDAR scan in one year and then we do it in another five years and we do inference on both of those point clouds,” Howe said.
“You can find the differences from that as well and see if there are more buildings, if any trees were cut down, and you can estimate the biomass changes.”
The research is just one example of the work resulting from AIML’s long-standing partnership with Lockheed Martin Australia (LMA), with LMA also sponsoring Howe’s attendance at the DICTA conference.
“Matt Howe's paper on improving the performance of point cloud segmentation models is just one example of how our strategic partnership with AIML is delivering machine learning research for national security, the space industry, business, and the broader community,” a Lockheed Martin spokesperson said.
As part of the strategic partnership, researchers from Lockheed Martin’s STELaRLab (Science Technology Engineering Leadership and Research Laboratory) work closely with AIML researchers in Adelaide, and support honours, doctoral and post-doctoral research and development programs.
For his PhD, which is partly sponsored by LMA, Howe is researching how computer vision can be used to improve road safety at intersections by investigating whether analysing trajectory data can help predict kinds of accidents and avoid near-misses.
“What we're trying to do is collect a whole lot of trajectory data, and then try and infer whether a certain particular kind of accident will occur at an intersection,” Howe said.
Effective Utilisation of Multiple Open-Source Datasets to Improve Generalisation Performance of Point Cloud Segmentation Models was accepted into The International Conference on Digital Image Computing: Techniques and Applications (DICTA) 2022.
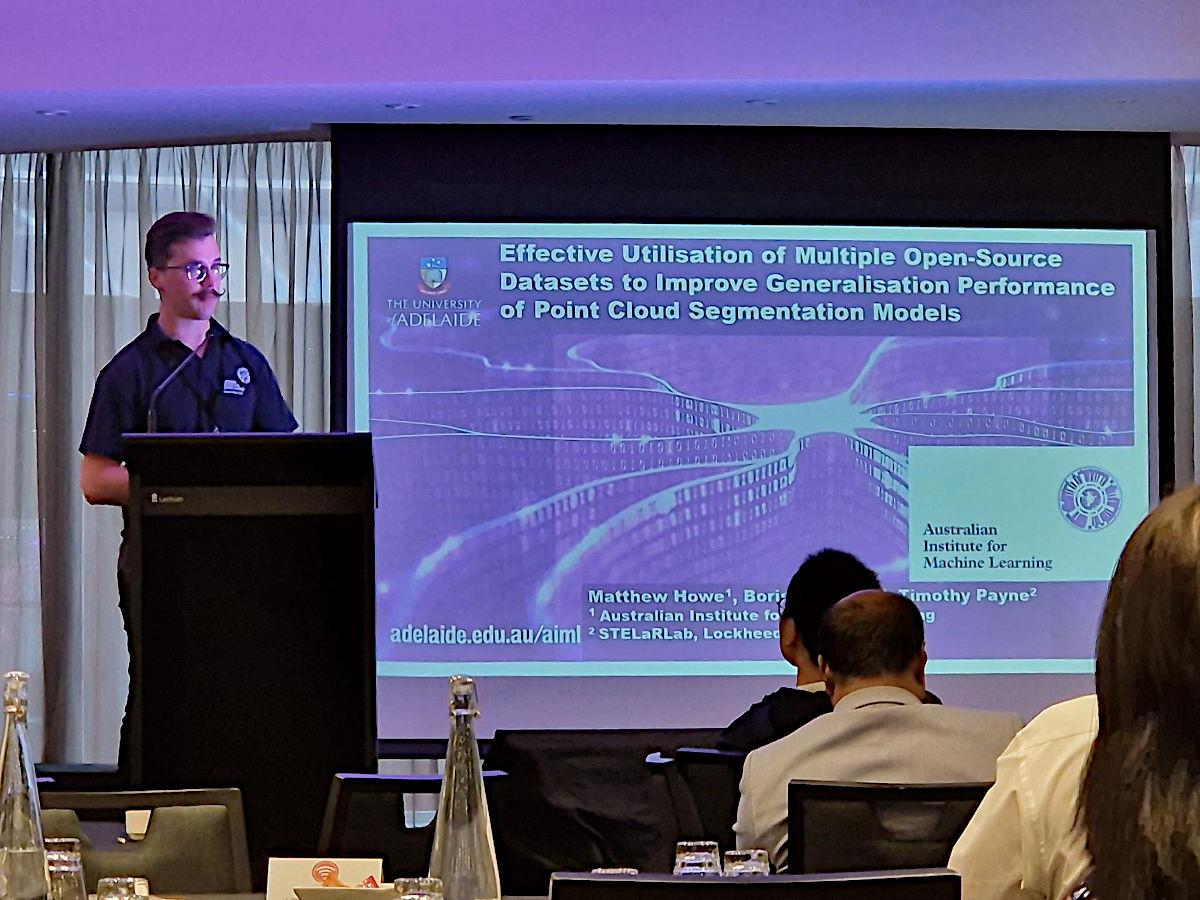
Matt Howe presenting his research at DICTA conference in Sydney.