Contextual awareness training for autonomous systems
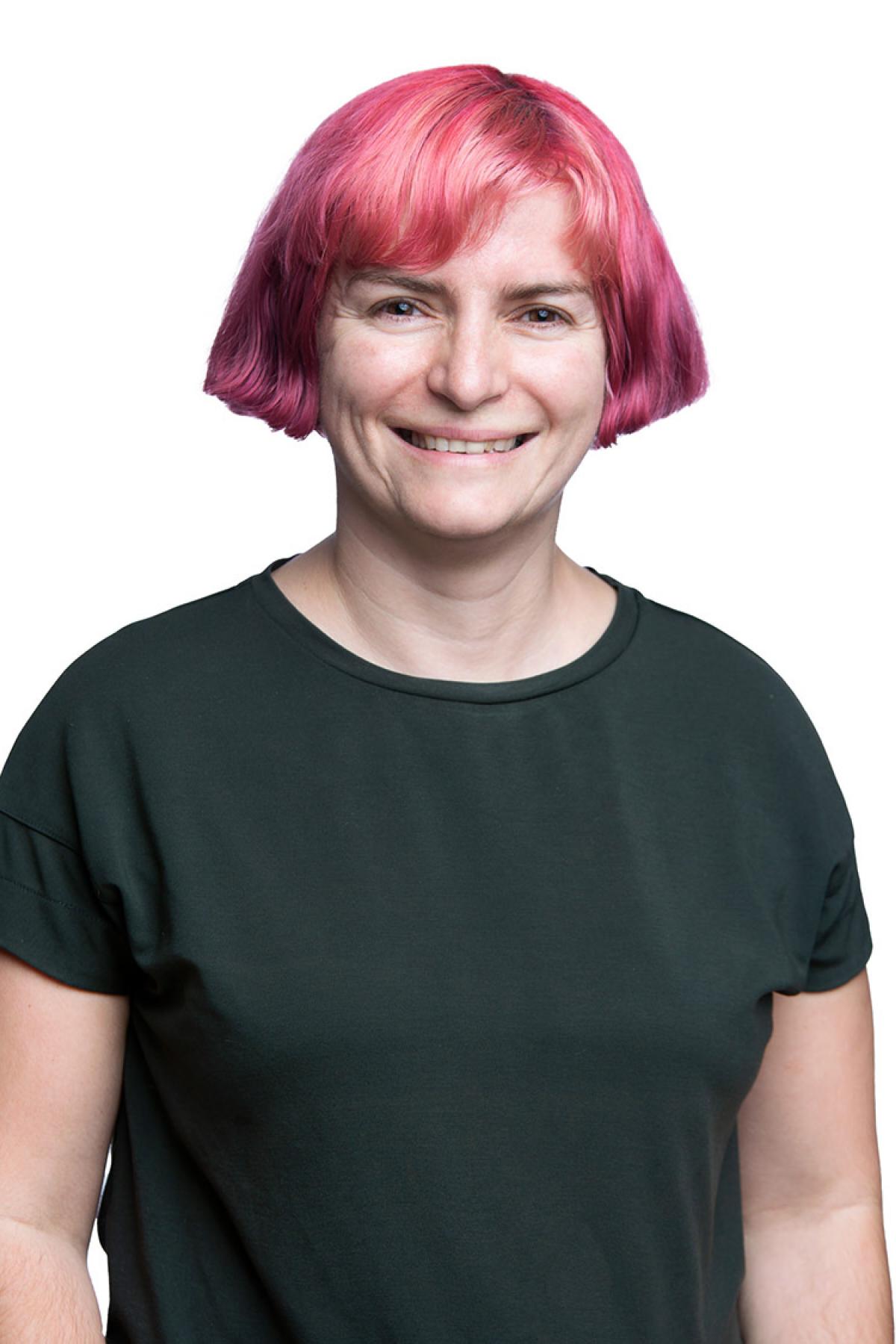
Associate Professor Claudia Szabo
Many algorithms used to train autonomous systems are based on variations of existing thresholds rather than truly unexpected new data.
Obviously, it is hard to know all the situations a system may encounter. Systems are also mostly trained in a laboratory environment where parameters can be easily controlled and maintained.
In the real-world however, the systems may find themselves under attack during operation, or simply encounter battery or connectivity issues which impact their ability to function effectively. To build complex, robust and resilient autonomous systems, we need to be able to train them to determine which course of action to take when the unexpected happens.
A/Prof Claudia Szabo and her team are looking at how we can create these increasingly resilient systems. Claudia explains "We need to be able to create algorithms which allow autonomous systems to determine what to do next even when this is outside what they have previously learned or experienced. My team is working to understand how we build these systems so they can effectively decide what information is relevant in a given situation and offer a quick and accurate response."
"It may be that the correct and only response is to bring the human operator in to the decision-making process, in which case we need to ensure the autonomous system can provide a quick, comprehensive, relevant and accurate understanding of what’s happening, and ideally a suggested response based on this analysis."
"We also need to make these learning and decision-making paths transparent and understandable to human operators. Trust between them and their autonomous counterparts is vital and you can’t trust what you can’t understand."
Claudia’s work not only has huge implications for the use of autonomous systems in the defence sector, but also offers huge benefits for emergency services who are increasingly using these systems in response to natural or manmade disasters.