Robust statistics
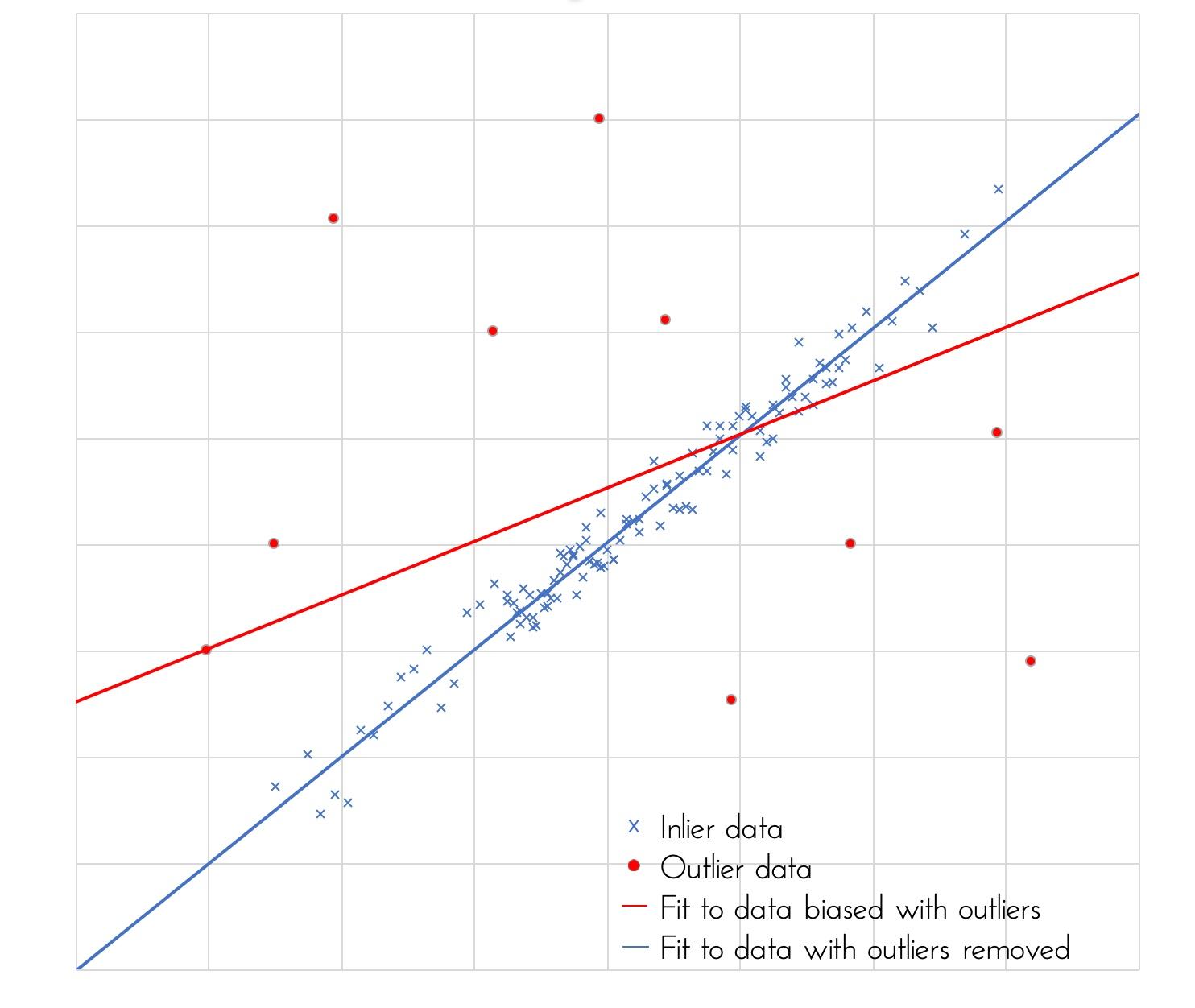
Machine learning algorithms rely on the ability to reconcile ideal mathematical models with real-life data that is noisy and contaminated by outliers. Robust statistics is a class of estimation techniques that identify patterns in this imperfect data, reducing the influence of smaller subgroups and outliers. Without robust statistical methods, data analysis can result in biased answers and conclusions.
AIML researchers have made significant contributions in advancing the theory and developing tools for robust statistics.
Robust statistics plays a central role in many computer vision applications. Outlier-contaminated data is a fact of life in computer vision. Data acquisition devices/sensors (imaging sensors, depth sensors, laser scanners, etc.) are inherently imperfect and cannot avoid making some spurious measurements. Also, the sensors seldom directly measure the quantity of interest and some form of pre-processing is required to extract the quantities of interest. This pre-processing step frequently introduces errors or outliers. For computer vision techniques to perform reliably and accurately in practical settings, the processing of the input data must be conducted robustly.
-
Featured publications
2017 “The Maximum Consensus Problem Recent Algorithmic Advances”
USA: Morgan & Claypool Publishers
Tat-Jun Chin, David Suter This book provides an overview of the algorithms for performing robust optimisation. The emphasis is on the basic operation or “inner workings” of the algorithms, and on their mathematical characteristics in terms of optimality and efficiency. The applicability of the techniques to common computer vision tasks is also highlighted. By collecting existing techniques in a single article, this book aims to trigger further developments in this theoretically interesting and practically important area.
2019 “Satellite Pose Estimation with Deep Landmark Regression and Nonlinear Pose Refinement” Bo Chen, Jiewei Cao, Álvaro Parra, Tat-Jun Chin We outline our award-winning monocular pose estimation framework for space-borne objects such as satellite. Our framework exploits the strength of deep neural networks in feature learning and geometric optimisation in robust fitting. In particular, the high-resolution representation of images used in HRNet enables accurate predictions of 2D landmarks while the SA-LMPE algorithm allows further removal of inaccurate predictions and refinement of poses. Our CV-based evaluation also indicates our method significantly outperforms previous work on the SPEED benchmark.
2019 "Star Tracking using an Event Camera" Tat-Jun Chi, Samya Bagchi, Anders Eriksson, Andre ́van Schaik
We investigate event cameras for star tracking. The main components in our processing pipeline are novel formulations of rotation averaging and bundle adjustment. We also developed a simulation technique for testing our method. Our results suggest that star tracking using event cameras is feasible and promising.
2018 “Guaranteed Outlier Removal for Point Cloud Registration with Correspondences” Álvaro Parra, Tat-Jun Chin An established approach for 3D point cloud registration is to estimate the registration function from 3D keypoint correspondences. Typically, a robust technique is required to conduct the estimation, since there are false correspondences or outliers.
We present a guaranteed outlier removal technique for robust Euclidean point cloud registration with correspondences. Any datum removed by our method is guaranteed to not exist in the globally optimal solution. Based on simple geometric operations, our algorithm is deterministic and efficient. Experiments show that, by significantly reducing the amount of data and outliers, our method greatly speeds up the solution (local and global) of the registration.
2015 “Guaranteed outlier removal for rotation search” Álvaro Parra, Tat-Jun Chin We propose a novel outlier removal technique for rotation search. Our method guarantees that any correspondence it discards as an outlier does not exist in the inlier set of the globally optimal rotation for the original data. Based on simple geometric operations, our algorithm is deterministic and fast. Used as a pre-processor to prune a large portion of the outliers from the input data, our method enables substantial speed-up of rotation search algorithms without compromising global optimality. We demonstrate the efficacy of our method in various synthetic and real data experiments.
2014 “Fast rotation search with stereographic projections for 3D registration” Álvaro Parra, Tat-Jun Chin, Anders Eriksson, Hongdong Li, David Suter Registering two 3D point clouds involves estimating the rigid transform that brings the two point clouds into alignment. We present a novel branch-and-bound (BnB) algorithm for 3D rotation search. The result is a fast and globally optimal rotation search algorithm. Experimental results demonstrate the superior efficiency of our algorithm for point cloud registration, owing to a faster rotation search kernel.
2014 “Fast rotation search for real-time interactive point cloud registration” Tat-Jun Chin, Álvaro Parra, Michael S Brown, David Suter
We propose an interactive user-assisted point cloud registration system for partially-overlapping 3D point clouds obtained from LIDAR scans. Our fast rotation search algorithm delivers globally optimal results in real time. Our method conducts branch-and-bound optimisation with a novel bounding function whose evaluation amounts to simple sorted array operations. Our system is intuitive and helps to accelerate the registration of multiple scans.
-
Projects
Space-Based Space Surveillance with Robust Computer Vision Algorithms
The Earth's orbit hosts Trillions of dollars worth of space assets such as communications and navigational satellites. Space utilisation is also rapidly increasing with participation from new government and civilian agencies. There is thus a pressing need for space environment monitoring, so as to protect valuable assets by preventing collisions and possibly covert sabotage. The project aims to develop computer vision algorithms to detect manmade objects in space. The algorithms are designed to function on nanosatellite platforms, thus enabling spacebased space surveillance. This resulting technology will provide always-on monitoring of the Earth's orbit to enhance existing defence infrastructure and protect vital space assets.
Tat-Jun Chin, Inovor
ARC Grant: LP160100495
Robust and accurate computer vision systems
The project aims to develop algorithms to support the development of robust and accurate computer vision systems. Real-world visual data (images, videos) is inherently noisy and outlier prone. To build computer vision systems that work reliably in the real world, it is necessary to ensure that the underlying algorithms are robust and efficient. The project aims to devise novel algorithms that can compute the best possible result given the input data in a short amount of time. The expected outcomes would support the construction of reliable and accurate computer vision-based systems, such as large-scale 3-D reconstruction from photo collections, self-driving cars and domestic robots.
Dr Tat-Jun Chin; Professor David Suter
ARC Grant: DP16010390
Lifelong Computer Vision Systems
The aim of the project is to develop robust computer vision systems that can operate over a wide area and over long periods. This is a major challenge because the geometry and appearance of an environment can change over time, and long-term operation requires robustness to this change. The outcome will be a system that can capture an understanding of a wide area in real time, through building a geometric map endowed with semantic descriptions, and which uses machine learning to continuously improve performance. The significance will lie in turning an inexpensive camera into a high-level sensor of the world, ushering in cognitive robotics and autonomous systems.
Ian Reid
ARC Grant: FL130100102
Computer vision from a multi-structural analysis framework
Computer vision has applications in a wide variety of areas: security (video surveillance), entertainment (special effects), health care (medical imaging), and economy (improved automation and consumer products). This project will improve the accuracy and reliability of such applications. Advances will also lead to new products and industries.
David Suter
ARC Grant: DP110103637
Statistical Methods of Model Fitting and Segmentation in Computer Vision
Electronic sensors such as cameras and lasers can provide a rich source of information about the position, shape, and motion of objects around us. However, to extract this information in a reliable, automatic, and accurate way requires a sophisticated statistical theory of the process. Example applications include: video surveillance (better automatic detection of moving people and vehicles and of characterising what those people and vehicles are doing), industrial prototyping and inspection (measuring the size and shape of objects), urban planning (laser scanning streetscapes to create computer models of cities), entertainment industry (movie special effects and games), etc.
Prof D Suter; A/Prof A Bab-Hadiashar
ARC Grant: DP0880553
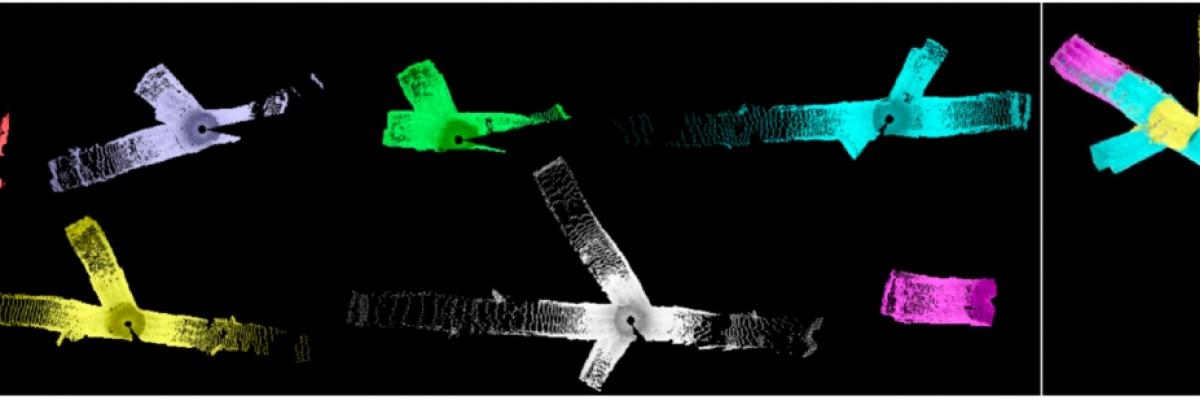
A set of partially overlapping 3D laser scans (viewed from the top) from an underground mine and their correct registration.